
AI in higher education: challenges, perceptions, and use cases
EDUCAUSE recently published some interesting survey data and analysis on what people in Higher Ed know and think about AI – and how (and if) they’re using it.
The conclusion is interesting: Higher Ed isn’t leveraging AI enough at the institutional level. “Current use of AI is a mile wide and an inch deep.”
It’s not difficult to see why. AI is an extremely nebulous concept, and often presented in such an abstract, future-oriented way that many struggle to understand it as something that can be concretely applied to their organization or their job right now.
A lot of institutional leaders don’t know whether or not they’re using AI
EDUCAUSE’s data reveals that a surprising number of institutional leaders don’t actually know the extent to which AI is deployed across various parts of their organization.
- 8% are unaware of the use of AI for instructional tasks (like plagiarism detection and tutoring.)
- 20% to 33% don’t know if AI is used for institutional tasks (like planning support resources and development and fundraising.)
- And 10-32% are unaware whether or not AI is being used for student success and support.
So what is AI? And how can we make use of it?
An “artificial intelligence” is simply a program that can simulate human intelligence and behaviour. Its ideal characteristic is the ability to rationalize data, and predict or formulate action plans similar to those a human would take in order to achieve specific goals.
Within AI we also have the concept of machine learning. Programs capable of machine learning can – within reason –learn from the patterns in data and act appropriately without any human intervention.
In Higher Ed, these capabilities present several obvious use cases: the ability to leave the processing of large amounts of data to computers can help to rationalize the back office, predict and respond to individual and group student performance, create bespoke tutoring programs, and spot plagiarism.
Sounds good. So what’s stopping us?
Even though there’s an awareness that AI can make institutions run more smoothly and give administrative staff a better workplace experience, the study identifies several common challenges facing institutions as they look to adopt more AI in their operations. Three of these are particularly important to us because we see them all the time, and because technology can do something to help.
AI adoption challenge 1: ineffective data governance, management, and integration
Nearly three-quarters (72%) of respondents said that ineffective data management and integration presents at least a moderate challenge to AI implementation.
This is certainly a problem, and the first one your institution will need to address. Bad data can only yield bad analysis or as they say in computing science: “garbage in, garbage out.” Poor integration will only make this problem worse.
If you’re struggling with governance, management, and integration, you probably need to consider advancing your digital transformation agenda sooner rather than later. Starting with the systems that create your institution’s operational foundations is the only way to ensure effective data management and capture, as no matter how much data you’re generating, only accurate, granular data that’s captured in a coherent way can generate accurate and actionable insights. Without a solid set of policies and processes at the capture stage, innovation will stagnate as it becomes impossible to coherently benchmark and track improvements.
Ironically, it’s often the case that this barrier to AI adoption can be overcome – at least in part – using AI itself. Using machine learning at the initial capture of transactional data can improve accuracy, which is foundational to better insights. For example, here at Unit4 we are currently using AI and ML in receipt recognition for travel expenses and incoming invoices to expedite and improve two of the most error-prone and time consuming processes many organizations face.
AI adoption challenge 2: Insufficient technical expertise
71% of respondents saw a lack of know-how as at least a moderate barrier to AI adoption. This can certainly seem like a chicken-and-egg problem, but again AI can actually help your institution to overcome its lack of AI expertise.
Because AI’s main job is to handle things humans used to do manually, it shouldn’t be a centralized function. Rather than a complicated central command system, institutional AI can be pervasive and distributed. And to some extent this is probably already the case in the applications of AI you may already use, like FAQ chatbots or financial aid assessment tools. You shouldn’t need to recruit an AI specialist in IT to automate other peoples’ jobs. Instead, you’re giving your people the opportunity to create automated solutions that help them to do their jobs more easily. Giving them the power to set up processes they know all too well that run automatically so they can save time and focus on more important things.
However, creating this kind of distributed AI ecosystem does have one big technical prerequisite – your systems need to be flexible enough to allow AI modelling in drag-and-drop, user friendly interfaces that don’t require any from-scratch coding. Your systems will also need the capability to help you integrate with other solutions and data sources to create a complete picture and pivot in order to deal with future unknowns. This kind of “microservices” architecture is exemplified well by the Microsoft Azure platform’s ability to make use of existing technologies as services within itself.
We’ve enabled this kind of functionality in our enterprise solutions – for example, our virtual assistant Wanda can be preconfigured through integrations with other systems to help people submit expenses or absences, or access performance insights through natural language interactions via chat applications they already use instead of having to log into finance and HR systems. We also use AI to automate incoming invoice registration, and our FP&A solution uses AI in budgeting and forecasting to find a “best fit” routine based on a multitude of possible options.
AI adoption challenge 3: Ethical concerns and algorithmic bias
Concerns about ethics related to AI use (68%) and concerns about algorithmic bias (67%) pose significant challenges to AI implementation.
This is an extremely important consideration, and we can’t brush it under the rug. AI can only learn from what it has experienced before, and if what it hears is already based on biased information, its conclusions will themselves be biased.
What we’ve learned at Unit4 is that the best use cases for AI are less about predicting or replacing human behaviour, and more about identifying which repetitive, time-consuming parts of your workflows can be predicted, suggested, and confirmed. Leaving users to accept, reject, or amend recommendations made by the AI so that your people have more time to engage creatively with the high value work they signed up to do.
Another benefit of encouraging a culture of AI use throughout your institution relates to our earlier point about giving your people the opportunity to create their own AI solutions. This democratization of technology and business processes can serve to help everyone better understand the most important components of their working environment rather than leaving it as a black box that only the IT team can make sense of. The user remains the person driving – they control the AI, and not the other way round.
How can AI make Higher Ed more efficient right now?
Here are just a few examples of how we at Unit4 are already using AI to create a better working environment for Higher Education administrations – enabling institutions to work more efficiently and, ultimately, deliver better student experiences.
- Chatbots enabled with natural language processing (NLP)
- Financial modelling driven by machine learning
- Expense forecasting
- Program profitability analysis
- Data anomaly detection
- Smart invoice and receipt recognition
And in the future, we‘ll roll out more capabilities in development in R&D, like smart student credibility rating based on open invoices, payment behavior, and other external sources.
The bottom line
The biggest challenge facing AI use for improved Higher Ed operations is that it isn’t officially part of anyone’s job description… yet. We’re learning that it can help us all do our jobs more productively. This means our culture need to evolve to prioritize and incentivize innovative AI driven by the people who know their processes best. And we need to ensure our foundational systems are flexible enough to enable this culture of smart working.
If you’d like more information, we’ve recently released findings from a survey of finance professional about Finance, AI and the future of decision making.
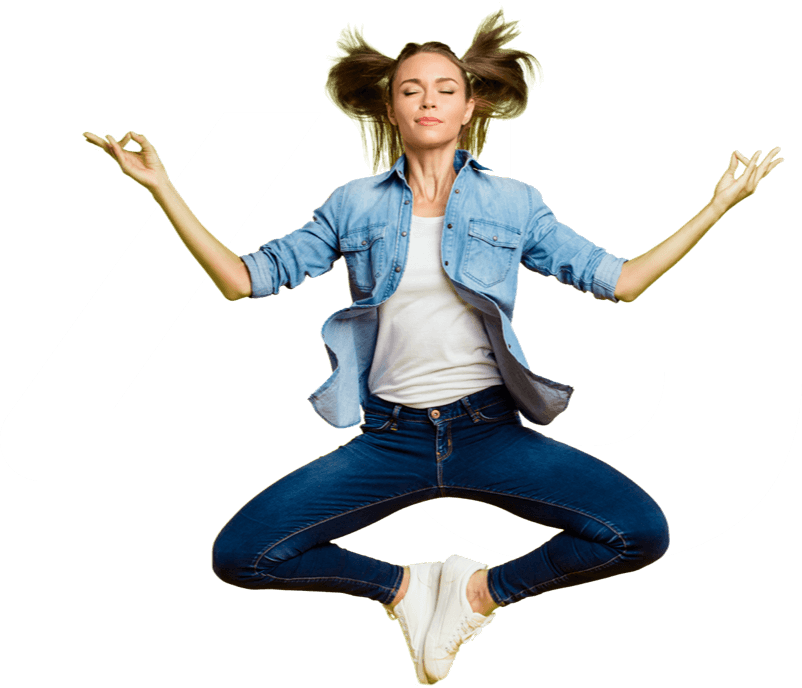